Recurve is excited to announce GRIDmeter 2.0, a major advancement toward accurately measuring the net impact of virtual power plants, demand response, energy efficiency, controllable devices, and other demand-side energy initiatives. This new version of the open-source GRIDmeter comparison methods utilizes a suite of machine learning methods, including advanced machine learning (ML) clustering algorithms, to enable significantly more robust measurement of net grid impact for portfolios and individual sites.
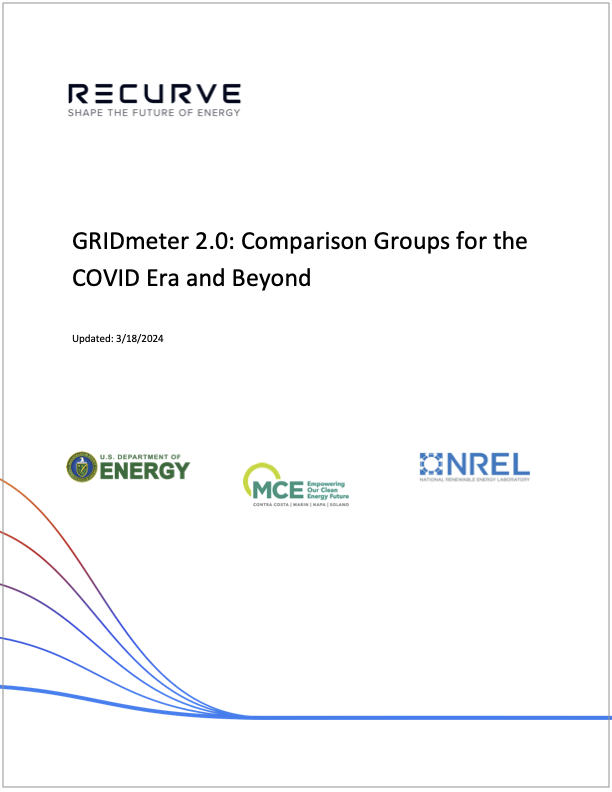
GRIDmeter 2.0 advances the state of the art for demand side resource measurement:
- Minimizes important differences between a treated site and its comparison group.
- Conducts matching using hourly model error rather than consumption.
- Applied to individual sites and aggregated to portfolios.
- Improves measurement stability by better utilizing the full comparison pool.
- In Recurve’s implementation, uncertainty is computed for any slice of results.
Increasingly accurate and transparent measurements of virtual power plants, energy efficiency, and demand response programs are critical to enable confidence in the resources, support grid integration, and provide fair compensation.
With so many factors influencing energy use, it is critical to consider the broader population alongside the changes experienced by participating customers. Was a customer saving because of more efficient lighting or because retail business slowed down? Is the response to an emergency event because of a DR program, or would it have existed anyway because people heard Flex Alerts on the radio?
To these ends, comparison groups generated by GRIDmeter 1.0 have been used successfully to measure dozens of demand-side programs, including energy efficiency, demand response, electrification, and electric vehicle charge-shifting initiatives. These measurements have proven effective even in extreme situations, such as COVID-19 and intense heat waves.
GRIDmeter 1.0 utilized “Individual Meter Matching,” considered an industry best practice. In this method, a comparison group is chosen from non-participants that share important categorical characteristics with a corresponding treatment group subpopulation (solar PV, climate zone, business type, etc.). With the available treatment and comparison group subpopulations in place, the individual meter matching procedure then selects the specific comparison group based on load shape similarity between each treatment meter and every possible comparison match. With the comparison group in place, changes in the comparison group are then propagated into the treatment group counterfactual to remove non-programmatic effects, yielding the net impact of the VPP or program.
While GRIDmeter 1.0 has proven very successful, Recurve has continued researching and testing new approaches that take advantage of modern machine learning and computational advancements and is proud to introduce Machine Learning (ML) Clustering, which improves several key aspects of comparison group selection and performance.
GRIDmeter 2.0 utilizes a suite of machine learning techniques to create “clusters” of non-participant meters with similar profiles, and each treatment meter is assigned an individualized correction based on a weighted assignment of clusters.

The next image gives an example of a treatment meter’s model error profile (bottom panel) and how the error patterns are matched by a weighted combination of clusters (top four panels). With the combination of clusters in place that best replicates the treatment meter’s baseline error profile, the comparison group corrections in the post-program period will have the greatest relevance. This process is done for every meter in the treatment group.
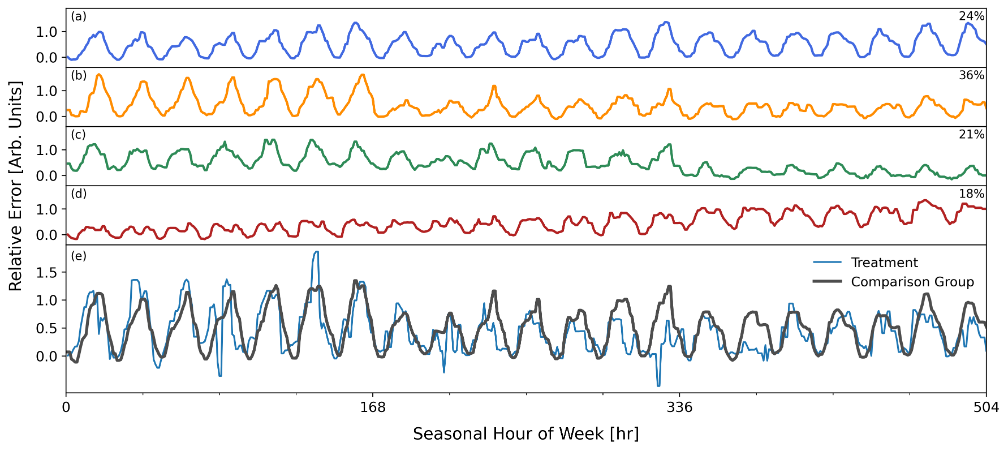
The ML Clustering approach has several key advantages:
- ML Clustering harnesses the full statistical power of the non-participant pool. With all suitable meters selected into a cluster, the comparison group takes advantage of the maximum statistical power available in the comparison pool. This trait of ML Clustering also mitigates the need for an evaluator to balance the size of the comparison group against the need for accurate load-shape matching, a tricky step that often requires judgment calls and is limited by the number of non-participants available.
- Clusters provide more stable and individualized comparison group corrections. In ML Clustering, every participant is given an individualized correction that depends on the trends observed in the unique weighted combination of clusters assigned to it. The individualized correction is far better for additional analyses that utilize meter-level results. In other comparison group methods, changing the composition of the participant sample will change the composition of a comparison group. In Individual Meter Matching, for example, adding a participant will add non-participant meters to the comparison group, thus changing the project results.
- Clusters are formed based on model error profiles, which are directly aligned with the goal of a comparison group. Comparison groups are intended to isolate and remove model errors. By formulating clusters based on baseline period model error profiles, matching can then be done on the metric that best reflects the ability of a comparison group to accomplish its objective. For instance, a participant who experienced a 25% reduction in consumption during the COVID period will exhibit a model error profile that reflects this change. That participant will be matched to clusters that best emulate that pattern. Many other selection methods focus on consumption or load shape matching, which are often correlated but ancillary to the main objective of the comparison group.
Through extensive testing, Recurve has optimized the GRIDmeter 2.0 ML Clustering algorithms and confirmed that the methods yield more accurate results with lower uncertainty than Individual Meter Matching.
Recurve has updated GRIDmeter to include ML Clustering, and these methods are now available through the open-source GRIDmeter repository. We have already used these methods to make the first successful measurement of PG&E’s Pilot Plus/Pilot Deep (a pilot building upon the Energy Savings Assistance Program), an initiative that will rely heavily upon trustworthy measurements in order to evolve and optimize both customer experiences and beneficial grid impacts.
The upgraded GRIDmeter 2.0 approach will be available to all Recurve customers — and as with many approaches, ML Clustering can continue to improve as we optimize with new datasets.
We look forward to deploying GRIDmeter 2.0 to enhance the quality and reliability of our measurements and serve our clients best!
Learn more about the GRIDmeter methods on the GRIDmeter website.