EVO Advanced M&V Testing Platform Results from the Recurve Platform, running the OpenEEmeter (CalTRACK 2.0)
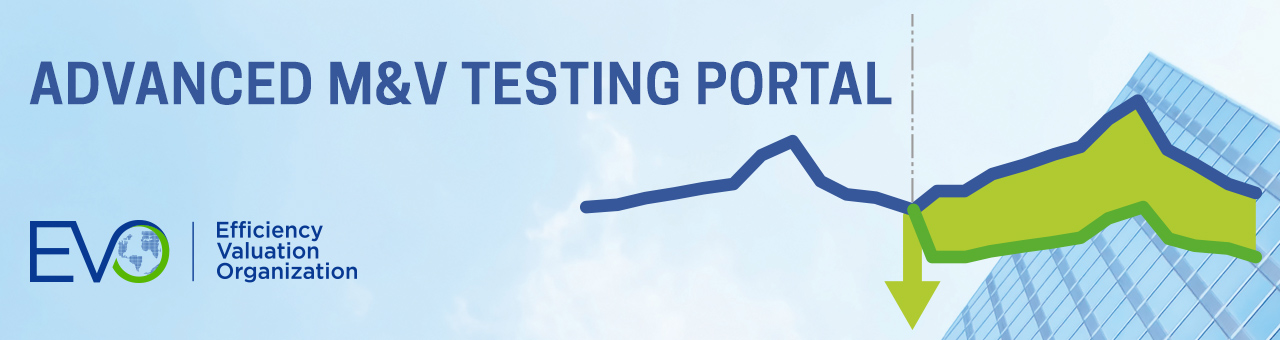
Results from the Efficiency Valuation Organization’s (EVO) Advanced M&V Testing Portal demonstrate that Recurve's implementation of the OpenEEmeter (2.6.0), running CalTRACK 2.0 hourly methods, is a strong contender for modeling commercial buildings.
CalTRACK 2.0 introduced an hourly model that is similar to LBNL’s open source Time-Of-Week and temperature (TOWT) model (www.caltrack.org). As part of the CalTRACK Methods process, the TOWT model was tested against a number of other potential modeling approaches using an out-of-sample testing process.
EVO’s testing portal allows users to compare Advanced M&V implementations and methods against each other using a single standardized dataset comprised of one year of hourly electricity data and outdoor temperature from 367 buildings from different regions in North America. According to EVO, “This testing service is founded upon a consistent methodology and industry-accepted performance metrics for M&V 2.0 software tools.”
The test results in two key metrics, the Coefficient of Variation of the Root Mean Squared Error, CV(RMSE), a measure of model precision and the Normalized Mean Bias Error, NMBE, which is a measure of overall model bias.

The Recurve Platform OpenEEmeter implementation performs on par with the LBNL Time of Week Temperature (TOWT) model, and better than all other models tested as of July 2019, including more complicated machine learning models based on decision trees and genetic programming. Compared to the LBNL TOWT model, the Recurve Platform OpenEEmeter running CalTRACK 2.0 performs slightly lower on average model precision and slightly better on average model bias. We would call it a tie.
In addition, CalTRACK stakeholders found that while the original LBNL TOWT model worked well for demand forecasting for commercial buildings, it did not perform as well on residential buildings. To address this problem, the CalTRACK process made modifications to the original TOWT model to capture a critical nuance of how energy is used in residential buildings--the fact that people use energy very differently at different times of the year. This nuance meant that LBNL TOWT model predictions were seasonally biased at different times of the year, even if the overall model was unbiased (see this CalTRACK issue for more details). These model updates vastly improved the CalTRACK model performance in residential buildings.
These test results indicate that CalTRACK 2.0 can continue to serve as a foundational open source measurement method for demand flexibility procurements and pay-for-performance programs in both residential and commercial buildings.
The Limits of Model Testing: A Case for a Different Approach
The EVO test provides a standardized test for a specific step in modeling building energy consumption. However, field performance for these models is subject to many other variables beyond just the modeling component.
While the CalTRACK model performs well with this testing approach, we feel that the approach itself does not necessarily represent real-world performance, for the following three reasons:
First, when it comes to calculating metered changes in energy consumption, data cleaning is half (or more) of the battle. Rules around issues like data sufficiency, interpolation, and weather station assignment can result in very different input data sets and thus generate quite different results. The CalTRACK process defines data cleaning explicitly (here), and Recurve provides a certificate that tests for compliance on each building included (here). If you don’t start with the same data, then your modeling is rather beside the point.
Second, the EVO test is designed to allow so called “black box” models to compare themselves to transparent models. Early results suggest that proprietary testers have been unwilling to reveal themselves (or uninterested in the test). Thus far, the anonymous models submitted have performed rather poorly. But even if they had performed well, in the real-world of meter-based performance, model transparency and reproducibility is a key acceptance criteria--a criteria made more critical as real money is often on the line, based on the results. As we learned in middle school math, you have to show your work to get credit.
Third, while EVO, LBNL and collaborators deserve credit for assimilating this initial 387-building data set, in order to set a standard bar for commercial buildings globally, the test must be expanded dramatically to cover more building types and climate zones and should differentiate performance for different classes.
Recurve recommends that models should be tested against real-world out-of-sample datasets based on a sample (or population) of buildings that have similar use types and locations as those for which the model will be deployed. The test should be expanded to include methods for data cleaning and eligibility and the handling of outlier buildings.
This type of out-of-sample testing can significantly improve model performance. For example, many of the improvements introduced in CalTRACK 2.0 were based on results from testing the CalTRACK 1.0 and OpenEEmeter on monthly data for over 50 million California meters. Open source collaboration where testing results are made transparent also drives significant modeling improvements through collaboration. (Learn more here.)
However, a one time test on a small sample of buildings, based on data that has already been cleaned and scrubbed of outliers, should not be confused with a certificate of “goodness” for any model, including CalTRACK or the OpenEEmeter.
Open Source is a better path forward for this industry.
Rather than continuing down the path of equivalency for disparate models and tools, Recurve believes the entire industry would benefit from collaboration on methods and code that supports the goal of scalability of EE as a resource, rather than the proprietary interest of software companies.
We invite all stakeholders to work together on the public CalTRACK Methods process to drive continuous improvement of the methods and jointly develop the OpenEEmeter Open Source implementation of these methods housed in the Linux Foundation (LF Energy).
Measurement cannot be owned by any entity and is where standard setting started. For efficiency to scale and engage markets and utility procurement, measurement must be open, transparent, reproducible, and free to all parties.

The efficiency industry wins when we stop struggling to justify software companies’ sunk costs and instead collaborate on consensus methods and open source code so everyone can be confident in their answers with nothing to hide.
Want to learn more about open source M&V for efficiency, procurement, and demand flexibility? Contact us.