Last fall, Mission:data, a nonprofit supporting energy data access, submitted comments to both the New Hampshire PUC and the New York PSC proceedings to simultaneously advocate for robust energy access and better privacy protections.
Do those two ideas sound contradictory?
They don’t have to be.
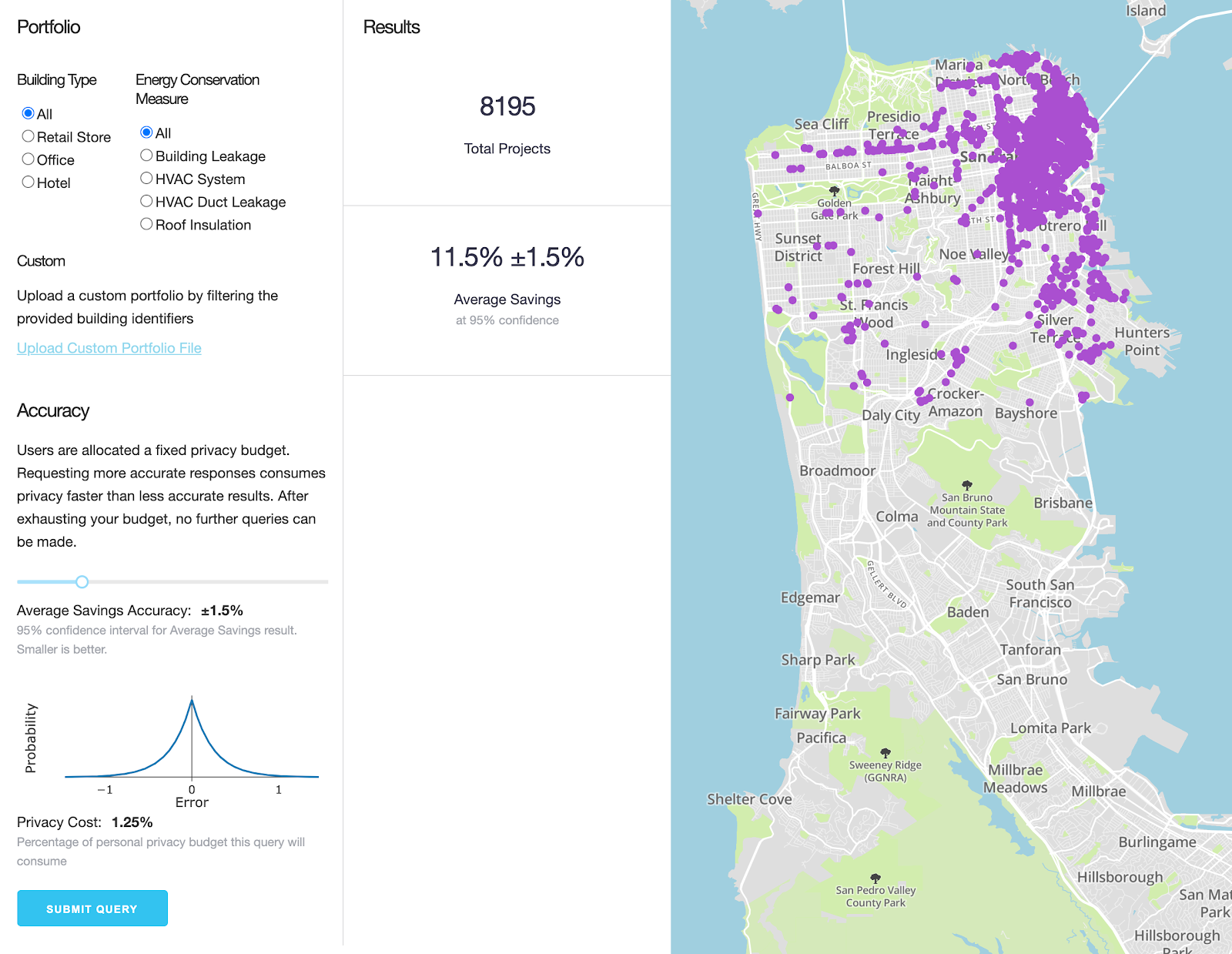
Recurve has been leading the development and implementation of rigorous methods, known as Energy Differential Privacy™ (EDP™), to enable the private and secure sharing of energy data outputs, in order to maximize its value for a range of public and private use cases. This multi-year effort was funded by the Department of Energy (DOE), with National Renewable Energy Lab (NREL), Lawrence Berkeley National Labs (LBNL), and the City of San Francisco. We are pleased to share the learnings and open source software that came from that effort.
We believe differential privacy will revolutionize our ability to put this valuable data to work while providing unprecedented, mathematically rigorous privacy protections to utility customers.
Many of the most valuable uses of energy data have historically been off-limits. Cities are developing plans to reduce carbon emissions without being able to actually calculate their footprints. Utility programs can’t tell the difference between a customer who needs a new AC system and one who would get the most out of lighting. Low-income programs are achieving lower than hoped impacts by treating the wrong customers. Cities are blind to the impacts of COVID on their citizens and business sectors.
The way to address all of these challenges is with better energy use data. But currently, third parties can usually only access consumption data that is pre-aggregated (meaning it can’t be queried and is therefore nearly useless) or anonymized to the point where it's unusable. Most often, they can't access it at all.
At the same time, while current simple aggregation schemes to protect customer privacy may suffice when using monthly data, these approaches fall apart when looking at AMI data and hourly impacts.
“Differential privacy” is an emerging best practice in which varying amounts of “noise” are added to aggregated statistics in order to mask any one individual’s contribution.
For example, the U.S. Census releases aggregated data about the U.S. population according to certain geographic areas known as “census blocks.” In a recent privacy assessment, the U.S. Census found that, in some census blocks, it was possible to re-identify as many as 46 percent of individual respondents’ race, ethnicity, sex, and age. Simply adjusting aggregation parameters was found to be insufficient to protect privacy, and differential privacy was chosen as the core privacy technology for the 2020 decennial census. Other applications of differential privacy include Google and Facebook’s analysis of detailed location data in tracking COVID-19 infections.
In our next blog, we’ll explain more about how differential privacy works and how it can expand the uses of energy data while dramatically reducing privacy risk compared to the status quo.
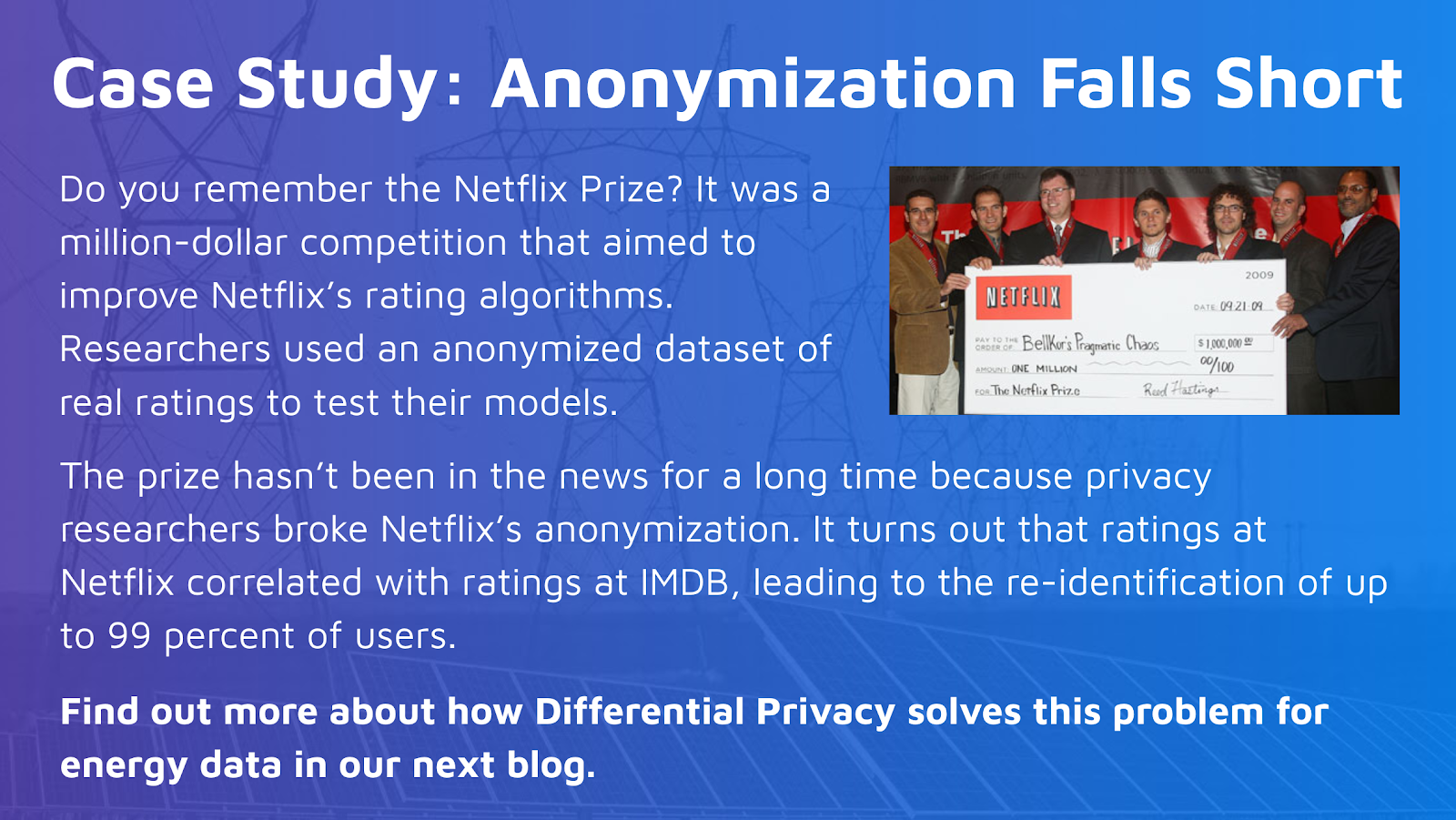
Read the Whole Series:
Part 1: Current Energy Data Privacy Methods Aren't Sufficient. We Have the Tools To Do Better.
Part 2: What Exactly is Energy Differential Privacy™? A Quick Tour of How it Works.
Part 3: Real World Use-Cases for Energy Differential Privacy™: Using EDP to Track COVID Impacts.
Learn more about Energy Differential Privacy™ on the the EDP website.
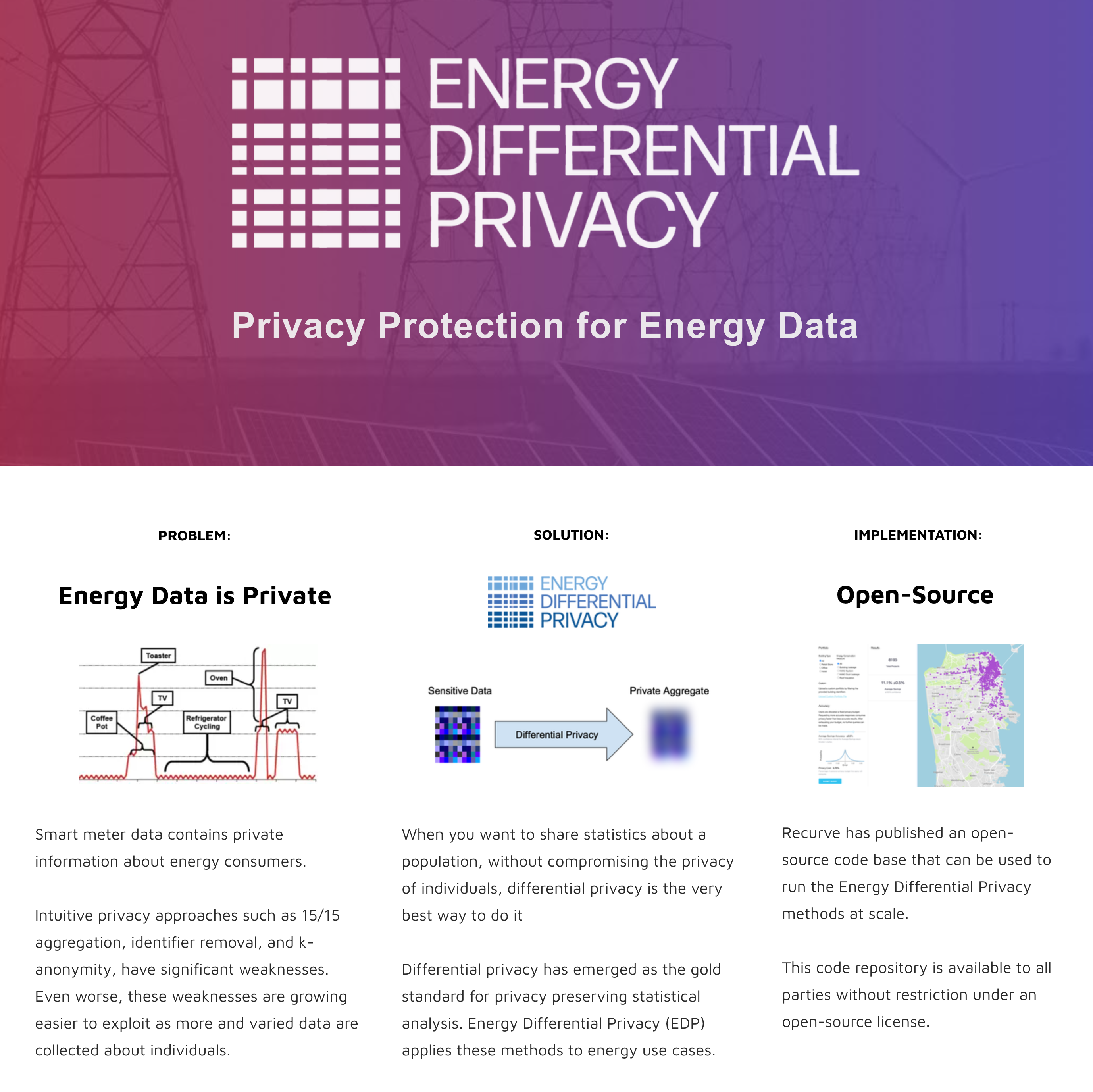